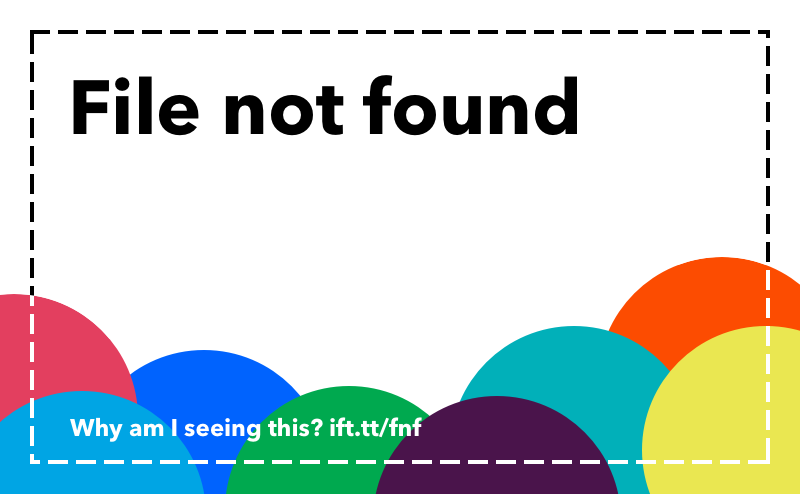
full image - Repost: Has anyone written comparing Hegelian dialectics to Bayes theorem? (from Reddit.com, Has anyone written comparing Hegelian dialectics to Bayes theorem?)
Mining:
Exchanges:
Donations:
Bayes theorem explains the optimal way to update a model from evidence, typically it is taught using simple models (i.e. a binary probability model) but in machine learning it is common to extend bayes theorem to more complicated probability models, included ones generated by neural networks. A lot of things can be represented as models, large language models like ChatGPT represent language as part of a generative model. Bayes theorem goes P(A|B) = P(B|A) * P(A) / P(B)P(A) is your initial model, which is kinda like a hypothesis. P(B) is your new evidence (which can also be a model, it gets complicated).P(B|A) is kind of like how well your old model explains the new evidenceAnd P(A|B) is your new model, incorporating the evidence of B into A. Then you repeat the process for any new piece of evidence you get.I'm starting to learn a bit more about Hegel and his dialectical method just keeps striking me as really related to this. P(A) = HypothesisP(B) = AntithesisP(A|B) = SynthesisP(B|A) is kinda tricky, but it's highly related to "How good of an argument against A is B" - i.e. does this new evidence (B) completely break your model A. I was searching around to see if anyone had written about this but google isn't getting me any hints. I figured if someone had then hopefully somebody here would know!
Social Media Icons